The Beautiful Relationship Between Machine Learning and Marketers
Loves Data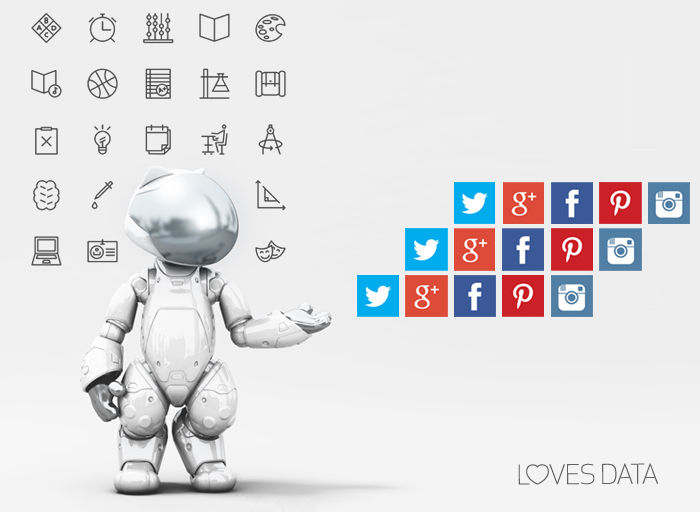
Machine learning’s been around for a while and it’s recently burst into popularity as a result of Google’s AI beating a human at their own game of ‘Go’ with a highly unorthodox move. It’s one of those things that I’m both terrified and complacent about. As a particularly lazy human, it’s a saving grace at the worst of times, but I’ve also watched Terminator, so I’m aware of how easily my pathetic flesh body is able to be squished by a machine. Back in reality, though, let's talk about how machine learning is helping digital marketers (warning: may contain mildly creepy overtones). To put a definition on it, machine learning is when a system recognises a pattern in behaviour and subsequently produces an output. Machine learning uses something called neural networks, which is a large system of software and hardware (that is capable of competing with the human brain). According to Wired’s apt analogy in their article about Elon Musk’s use of machine learning and neural networks in a Tesla; “Feed enough photos of a cat into a neural net, and it can learn to recognise a cat. Feed it enough human dialogue, and it can learn to carry on a conversation. Feed it enough data on what cars encounter while driving down the road and how drivers react, and it can learn to drive…”
Smart Goals
Teaching machines how to recognise cats, languages or to drive, not only helps the consumer, it also helps the advertiser to automate simple processes, so that they can (potentially) move to a more strategic level of marketing management. Take AdWords Smart Goals for example. Smart Goals are kind of like an ‘auto conversion’ tool for advertisers who are yet to set up an actual conversion. It doesn’t just pull the conversion out of thin air – Smart Goals use machine learning to determine who would convert based on signals like, sessions, location, device, and time spent on the page. Every visit is given a score and the ‘best’ visit is used to determine what a Smart Goal is. Best visits are seen as the top 5% of traffic coming from your AdWords campaigns and is then applied to every website visit (even those from other traffic sources).
Social Networks
Social networks are big fans of machine learning. You’ll never get to see the algorithm doing it, but your news feed is based on things you find interesting, plus content that has high engagement with the people you interact with or pages you follow. For example, Pinterest’s Pinability score uses machine learning so that pins in your feed appear in terms of relevance as opposed to chronologically. The team behind Pinability uses programs such as Logistic Regression (LR), Support Vector Machines (SVM), Gradient Boosted Decision Trees (GBDT) and Convolutional Neural Networks (CNN) as well as testing a bunch of text and image features to determine the Pinnability score. After that, A/B tests are performed on users to accurately determine the performance of pins, which is good if you’re posting popular pins which people are engaging with.
Other social channels that use relevance to structure their news feed are Facebook, Twitter and now Instagram. It’s arguably so brands are forced to pay for advertising space, but also to prevent users from being fed useless content and drowning in an avalanche of information overload. The algorithms are still learning, though. Facebook recently presented me with a ‘survey’ that had me choosing between two separate, but slightly similar posts from pages that I followed – a small reassurance that we’re still teaching the machines.
Hootsuite’s ‘suggested Tweets’ is an awesome example of how marketers can use machine learning to their advantage. Here are a few that were suggested to Loves Data:
- “Google launches Trial Run Ads for apps in search results to let users play before installing http://ow.ly/3cqWUJ”
- “Go board game champion Lee Sedol finally beats Google’s AI http://ow.ly/3cqWUL” – which is literally a suggested Tweet about machine learning.
- “Google beefs up ads, analytics, and updates for Android game developers http://ow.ly/3cqWUM”
All of these ‘suggested Tweets’ are very much on brand with Loves Data’s content, and the sources are all from websites we follow avidly. This means we’ve been ‘feeding Hootsuite enough cats’ for them to understand and then predict the kind of websites that Loves Data (and therefore our audience) would be interested in Tweeting. Hootsuite also has a new app which suggests your messages, showing that they're able to use machine learning to create actual, complex sentences.
Perhaps one of the more obvious examples of social media using machine learning is Facebook (or Google+’s) ability to recognise faces. People have developed machines that are able to produce their own outcomes, without having to state anything. And it’s surprisingly effective – Facebook’s able to recognise almost anyone with an account and suggest, very accurately, who they are. And now, Facebook’s AI is automatically writing photo captions for people who have vision impairments. It’s using a tool called Automatic Alternative Text which uses deep neural networks to determine what particular objects within photos are, for example, a chair or table, as well as reading meaning into people’s body language. Although this technology isn’t 100% spot-on yet (it still can’t write complete sentences), it gives us a glance into what the future holds.
Using Machine Learning to make User Predictions
The Google Prediction API is a great example of how pattern matching and machine learning can be used to analyse your data to make predictions. It’s a cloud-based API and once it’s ‘learned’ from your data, it predicts a number or category to describe your data. This means you can create applications that will show what products your customer might be interested in, classify your emails or predict how people will spend in a given day. Sound interesting? You can get started with it here.
There’s also a really cool tutorial over at Your Cloud Buddy, which shows you how you can load Google Analytics into R and use machine learning to predict user behaviour. The tutorial uses the example of being able to predict a user’s browser via their region. By creating a One Rule model, you can use the nth minute, region and browser dimensions, with hits by the minute as the metric. Check it out!
Email Marketing
Speaking of machine learning taking over online human communications, Litmus recently asked more than 1,100 marketers “Will machine learning, AI and predictive software ever determine the majority of the content (subject lines, images, copy etc.) in marketing emails?” About 66% of the selected group said ‘yes!’
However, according to Marketing Land, there are a couple of areas that humans excel in, where machines will fall short. Things like setting business objectives, being creative and handling events that are out-of-the-ordinary are still intrinsically human. It’s predicted instead, that whilst machine learning within email marketing could work within templates (e.g. pulling headings and description text) people will still manage any other tasks that require a degree of emotion.
People have been writing, predicting, analysing and criticising machine learning for years (because we’re excited and terrified at the same time). If you’re interested in the topic, we recommend checking out Google Search will be Your next Brain by Steven Levy. It’s an epic 21-minute read about how Google uses machine learning and artificial intelligence within its advertising, self-driving cars, search engines and even medicine. Although it’s from early 2015, it’s still super helpful to gain an understanding of what machine learning means and its implications for humanity.
What are your thoughts on machine learning and its impact on marketing? Let us know in the comments section.
Comments